Research
WORKING PAPERS
- Miao, Y., He, Q., Saffarizadeh, K., & Kim, S. “When Should AI Challenge Us? Designing AI Feedback to Break the AI Echo Chamber in Human-AI Creative Collaboration,” Submitted
- Wang, P., Zefeng, B., Saffarizadeh, K., & Wang, C. “The Impact of App Updates on Usage Frequency and Duration,” Under 2nd round review
- Miao, Y., He, Q., Kim, S., & Saffarizadeh, K. “Creative Gains, Reputational Strains: Generative AI Elevates Style and Aesthetic Quality but Triggers Spillovers on Non-AI Artworks,” In preparation for a 2nd round review
- Arnold, C., Zhiming, X., Saffarizadeh, K., & Madiraju, P. “Generative AI as (Un)welcome Agents in Medical Crowdfunding: The Trust Dilemma and Moral Hazard,” Under 3rd round review
- Saffarizadeh, K., & Keil, M. “Conversational AI Agents: The Effect of Process and Outcome Variation on Anthropomorphism and Trust,” Under 4th round review
Relationship Between Trust in the AI Creator and Trust in AI Systems: The Crucial Role of AI Alignment and Steerability
Saffarizadeh, Kambiz; Keil, Mark; and Maruping, Likoebe (2024)
Journal of Management Information Systems (JMIS)
This paper offers a novel perspective on trust in artificial intelligence (AI) systems, focusing on the transfer of user trust in AI creators to trust in AI systems. Using the agentic IS framework, we investigate the role of AI alignment and steerability in trust transference. Through four randomized experiments, we probe three key alignment-related attributes of AI systems: creator-based steerability, user-based steerability, and autonomy. Results indicate that creator-based steerability amplifies trust transference from AI creator to AI system, while user-based steerability and autonomy diminish it. Our findings suggest that AI alignment efforts should consider the entity with which the AI goals and values should be aligned and highlight the need for research to theorize from a triadic view encompassing the user, the AI system, and its creator. Given the diversity in individual goals and values, we recommend that developers move beyond the prevailing ‘one-size-fits-all’ alignment strategy. Our findings contribute to trust transference theory by highlighting the boundary conditions under which trust transference breaks down or holds in the emerging human-AI environment.
Keywords: AI Alignment Problem, AI Trust, Trust Transference, Creator-Based Steerability, User-Based Steerability, AI Autonomy, Algorithmic Decision Making, AI Ethics
Privacy Concerns and Data Donations: Do Societal Benefits Matter?
Alashoor, Tawfiq; Keil, Mark; Jiang, Zhenhui; and Saffarizadeh, Kambiz (2025)
MIS Quarterly (MISQ)
Data donations, where individuals are encouraged to donate their personal information, have the potential to advance medical research and help limit the spread of pandemics, among other benefits. The decision to donate data is fundamentally a privacy decision. In this research, we build on the privacy calculus, a model describing privacy risks and benefits, and examine the impact of privacy concerns on data donation decisions, highlighting the role of societal benefits in privacy decisions. Based on two randomized experiments using the general context of data donation for medical research (Experiment 1) and the specific context of data donation for COVID-19 research (Experiment 2), we find that individuals who are highly concerned about privacy tend to donate less data (Experiments 1 and 2). This effect holds under a variety of conditions and is consistent with prevailing research. However, this effect is contingent on the privacy calculus. When implicit or explicit societal benefits are perceived, particularly in the absence of privacy controls, the association between privacy concerns and data donation decisions is less salient, highlighting the significant role that societal benefits play in privacy decisions. We discuss the theoretical, practical, social, and ethical implications of these findings.
Keywords: Privacy concerns, data donation, public health, privacy calculus, information disclosure, COVID-19, societal impact, behavioral experiment
My Name is Alexa. What’s Your Name?
The Impact of Reciprocal Self-Disclosure on Post-Interaction Trust in Conversational Agents
Saffarizadeh, Kambiz; Keil, Mark; Boodraj, Maheshwar; and Alashoor, Tawfiq (2024)
Journal of the Association for Information Systems (JAIS)
The use of conversational AI agents (CAs), such as Alexa and Siri, has steadily increased over the past several years. However, the functionality of these agents relies on the personal data obtained from their users. While evidence suggests that user disclosure can be increased through reciprocal self-disclosure (i.e., a process in which a CA discloses information about itself with the expectation that the user would reciprocate by disclosing similar information about themself), it is not clear whether and through which mechanism the process of reciprocal self-disclosure influences users’ post-interaction trust. We theorize that anthropomorphism (i.e., the extent to which a user attributes humanlike attributes to a nonhuman entity) serves as an inductive inference mechanism for understanding reciprocal self-disclosure, enabling users to build conceptually distinct cognitive and affective foundations upon which to form their post-interaction trust. We found strong support for our theory through two randomized experiments that used custom-developed text-based and voice-based CAs. Specifically, we found that reciprocal self-disclosure increases anthropomorphism and anthropomorphism increases cognition-based trustworthiness and affect-based trustworthiness. Our results show that reciprocal self-disclosure has an indirect effect on cognition-based trustworthiness and affect-based trustworthiness and is fully mediated by anthropomorphism. These findings conceptually bridge prior research on motivations of anthropomorphism and research on cognitive and affective bases of trust.
Keywords: Conversational AI, AI Agent, Chatbot, Cognition-Based Trust, Affect-Based Trust, Anthropomorphism, Reciprocal Self-Disclosure
Amanda Project
Amanda is a custom-designed conversational assistant that I developed to explore human-AI interaction. The project includes several studies, each focusing on a specific aspect of this interaction. Amanda comprises an Android app paired with a backend administration website. This website not only remotely controls the app but also integrates machine learning and artificial intelligence capabilities to enhance the app’s functionality.

HelpAIGrow Android App
Description: HelpAIGrow is a conversational assistant designed to help researchers study human-AI interaction. The app is available on Google Play Store.
Language: Java
Licence: GPLv3
Source Code: https://github.com/saffarizadeh/HelpAIGrow

HelpAIGrow Researcher Dashboard
Description: HelpAIGrow Researcher Dashboard is a server-side software that communicates with HelpAIGrow app. The dashboard enables the researchers to create and customize several types of experiments for the app.
Language: Python
Licence: GPLv3
Source Code: https://github.com/saffarizadeh/HelpAIGrowDashboard
Co-Authors
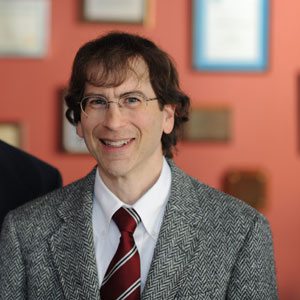
Mark Keil
Georgia State University
Personal Website
Google Scholar
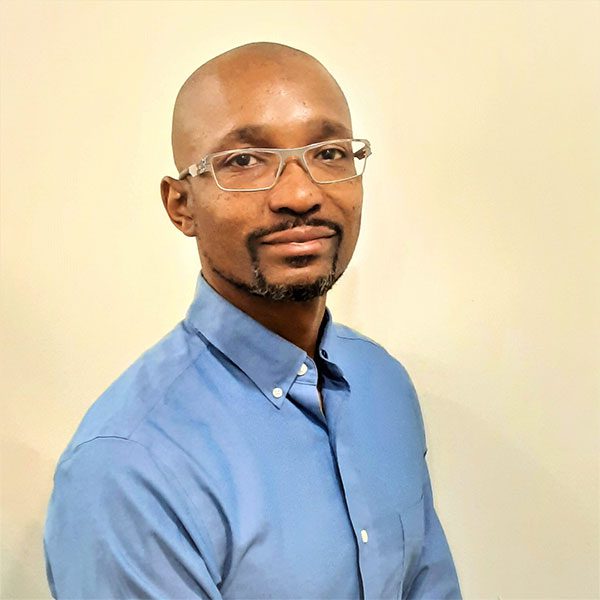
Likoebe M. Maruping
Georgia State University
Personal Website
Google Scholar
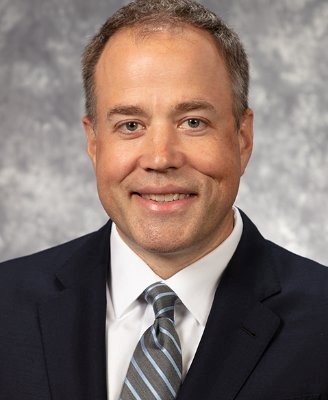
Nicholas Berente
University of Notre Dame
Personal Website
Google Scholar
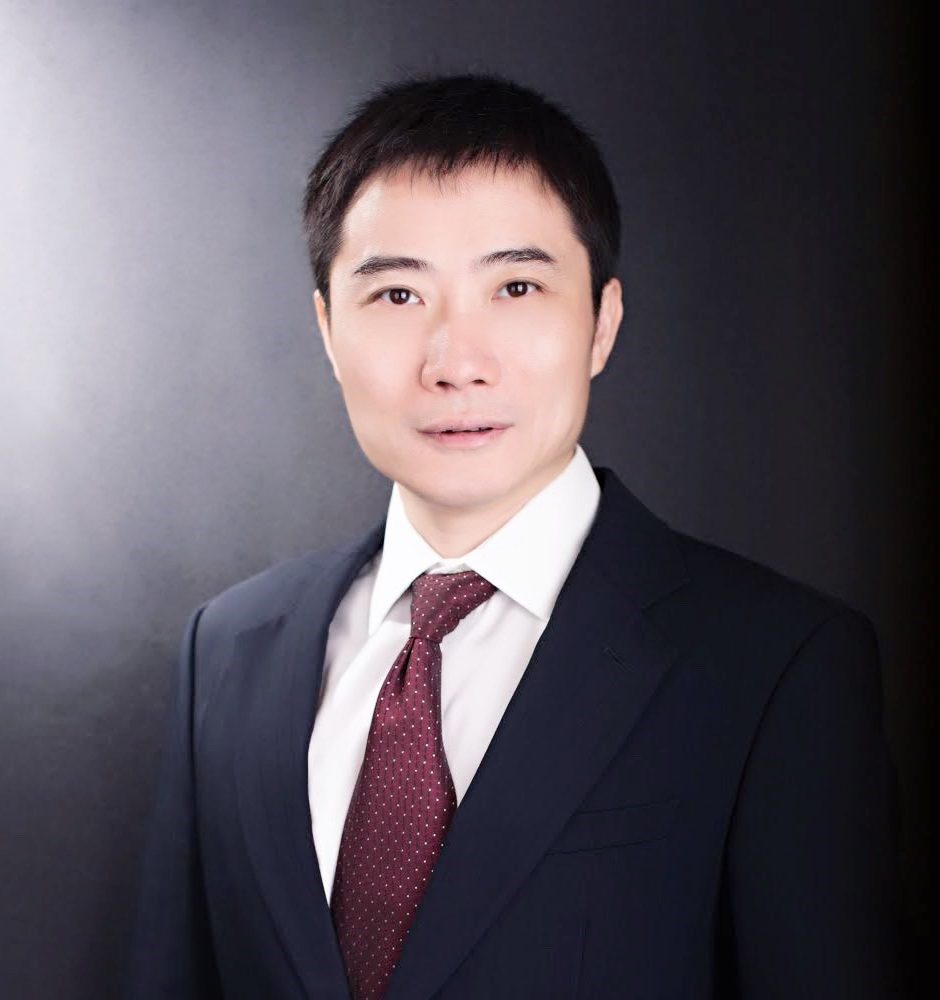
Zhenhui (Jack) Jiang
HKU Business School
Personal Website
Google Scholar
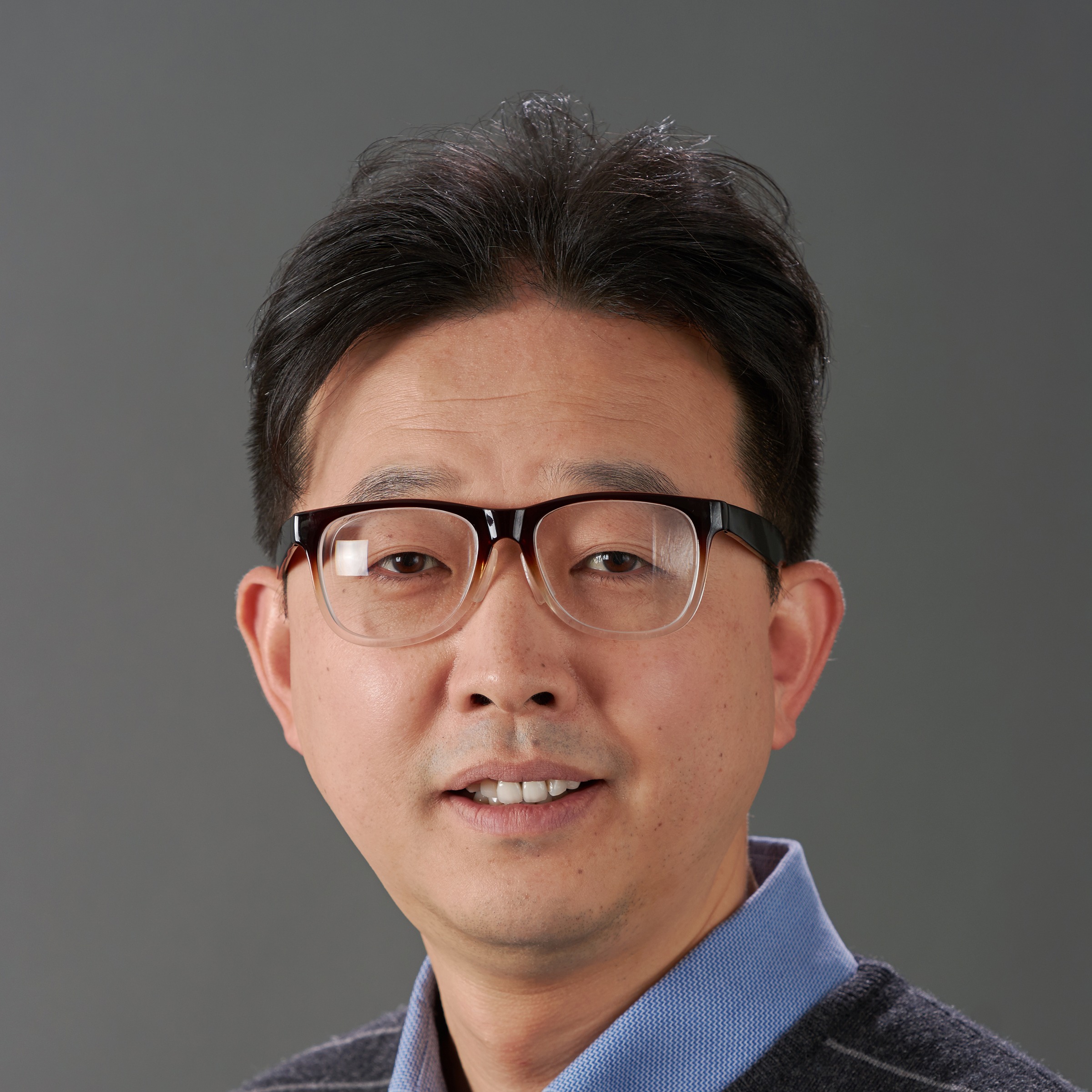
Sung S Kim
University of Wisconsin-Madison
Personal Website
Google Scholar
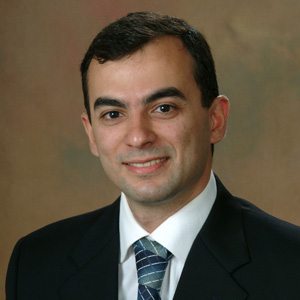
Wael Jabr
Penn State University
Personal Website
Google Scholar
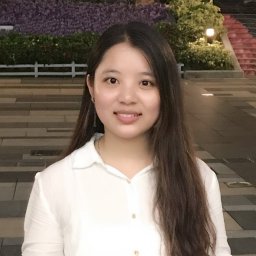
Yumeng Miao
University of Wisconsin-Madison
Personal Website
Google Scholar
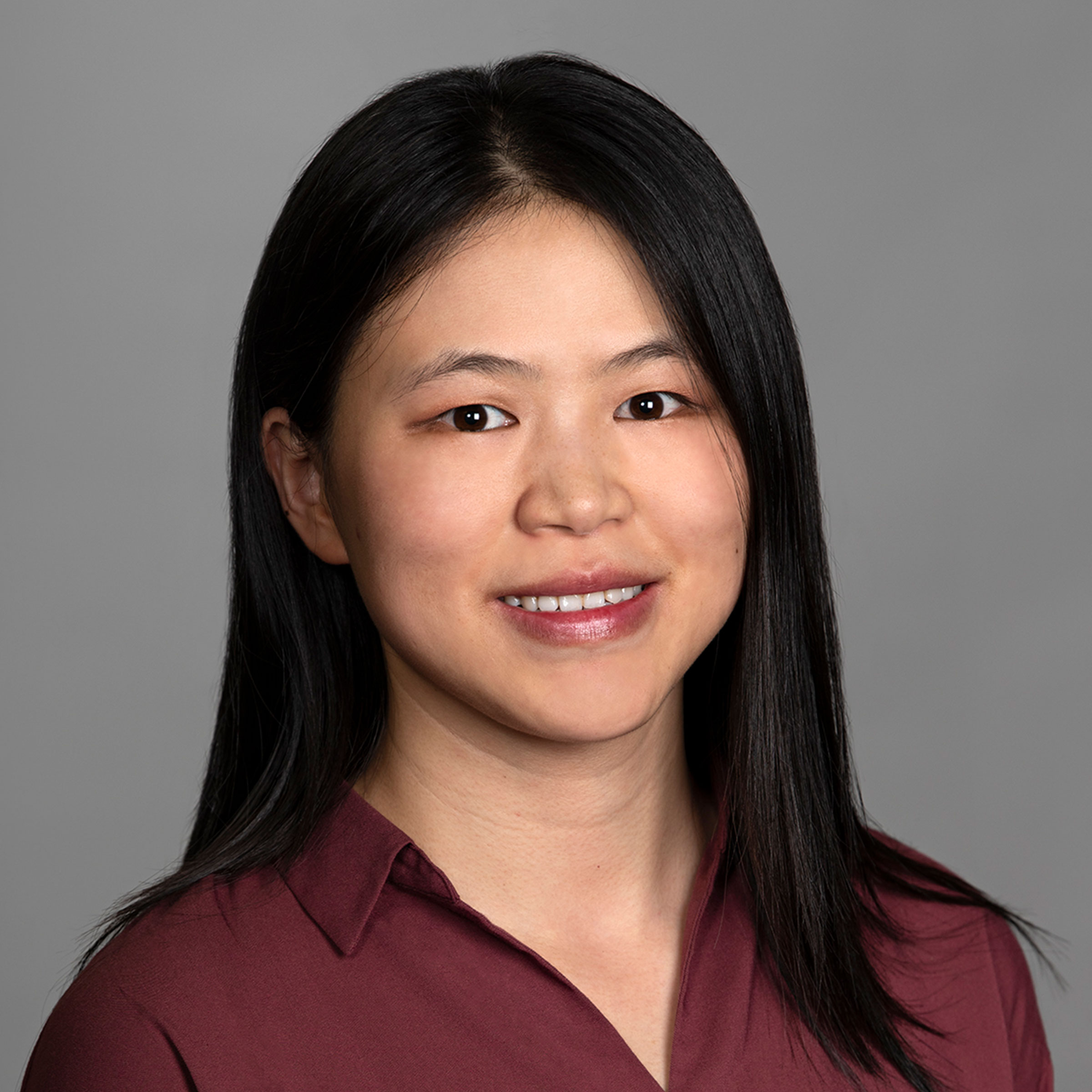
Qinglai He
University of Wisconsin-Madison
Personal Website
Google Scholar
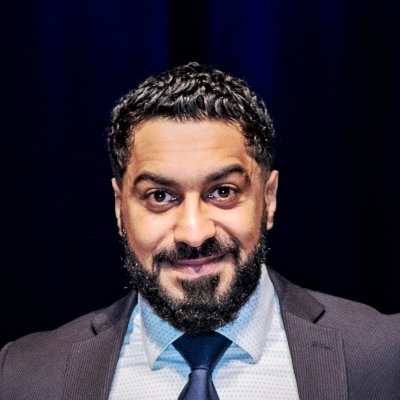
Tawfiq Alashoor
IESE Business School
Personal Website
Google Scholar
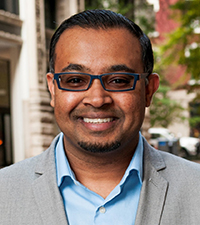
Maheshwar Boodraj
Boise State University
Personal Website
Google Scholar
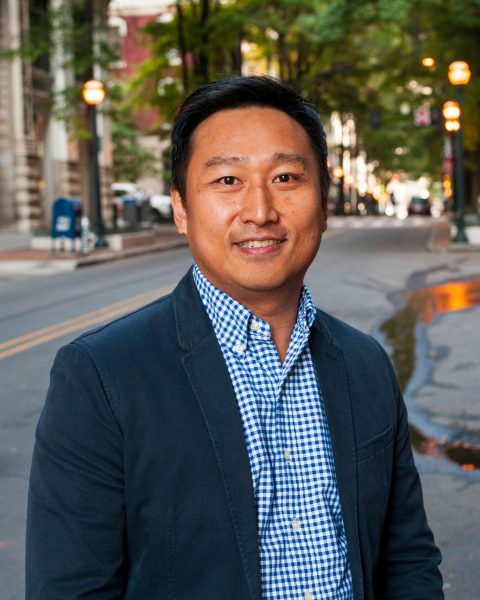
Hyoungyong Choi
Hankuk University of Foreign Studies
Personal Website
Google Scholar
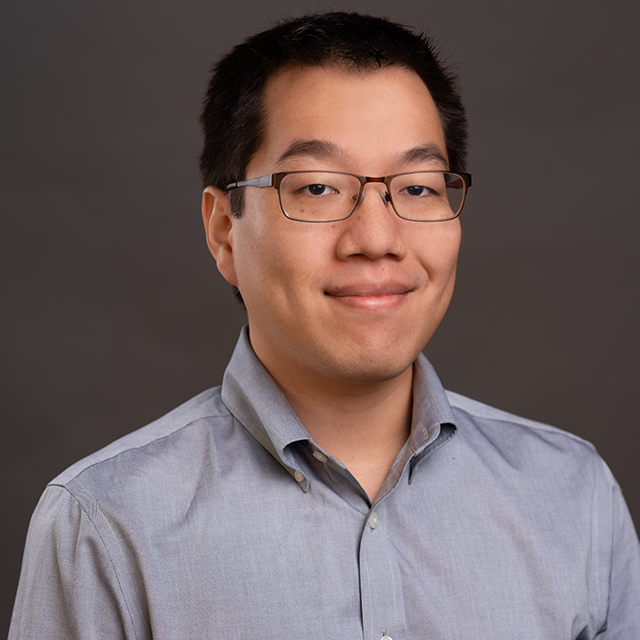
Alan Yang
University of Nevada, Reno
Personal Website
Google Scholar